Quick sort Python的問題,透過圖書和論文來找解法和答案更準確安心。 我們找到下列懶人包和總整理
Quick sort Python的問題,我們搜遍了碩博士論文和台灣出版的書籍,推薦Rioux, Jonathan寫的 Data Analysis with Python and Pyspark 和Adams, Thomas A.的 Learn Aspen Plus in 24 Hours, 2e都 可以從中找到所需的評價。
另外網站Hoare's Quicksort Algorithm in Python也說明:Quicksort overview · Quicksort sets the low and high partition indices · Pointer ( i ) travels from low up until pivot < array[i] · Pointer ( j ) travels from high ...
這兩本書分別來自 和所出版 。
最後網站An Overview of QuickSort Algorithm - Towards Data Science則補充:Quicksort is one of the most popular sorting algorithms that uses ... Let's look at quicksort programs written in JavaScript and Python ...
Data Analysis with Python and Pyspark
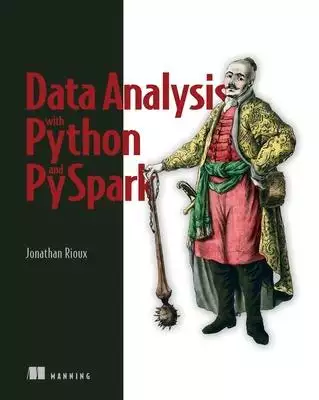
為了解決Quick sort Python 的問題,作者Rioux, Jonathan 這樣論述:
Think big about your data! PySpark brings the powerful Spark big data processing engine to the Python ecosystem, letting you seamlessly scale up your data tasks and create lightning-fast pipelines.In Data Analysis with Python and PySpark you will learn how to: Manage your data as it scales acros
s multiple machines Scale up your data programs with full confidence Read and write data to and from a variety of sources and formats Deal with messy data with PySpark’s data manipulation functionality Discover new data sets and perform exploratory data analysis Build automated data pipelines that t
ransform, summarize, and get insights from data Troubleshoot common PySpark errors Creating reliable long-running jobs Data Analysis with Python and PySpark is your guide to delivering successful Python-driven data projects. Packed with relevant examples and essential techniques, this practical book
teaches you to build pipelines for reporting, machine learning, and other data-centric tasks. Quick exercises in every chapter help you practice what you’ve learned, and rapidly start implementing PySpark into your data systems. No previous knowledge of Spark is required. Purchase of the print boo
k includes a free eBook in PDF, Kindle, and ePub formats from Manning Publications. About the technology The Spark data processing engine is an amazing analytics factory: raw data comes in, insight comes out. PySpark wraps Spark’s core engine with a Python-based API. It helps simplify Spark’s steep
learning curve and makes this powerful tool available to anyone working in the Python data ecosystem. About the bookData Analysis with Python and PySpark helps you solve the daily challenges of data science with PySpark. You’ll learn how to scale your processing capabilities across multiple machin
es while ingesting data from any source--whether that’s Hadoop clusters, cloud data storage, or local data files. Once you’ve covered the fundamentals, you’ll explore the full versatility of PySpark by building machine learning pipelines, and blending Python, pandas, and PySpark code. What’s inside
Organizing your PySpark code Managing your data, no matter the size Scale up your data programs with full confidence Troubleshooting common data pipeline problems Creating reliable long-running jobs About the reader Written for data scientists and data engineers comfortable with Python. About th
e author As a ML director for a data-driven software company, Jonathan Rioux uses PySpark daily. He teaches the software to data scientists, engineers, and data-savvy business analysts. Table of Contents 1 Introduction PART 1 GET ACQUAINTED: FIRST STEPS IN PYSPARK 2 Your first data program in PySp
ark 3 Submitting and scaling your first PySpark program 4 Analyzing tabular data with pyspark.sql 5 Data frame gymnastics: Joining and grouping PART 2 GET PROFICIENT: TRANSLATE YOUR IDEAS INTO CODE 6 Multidimensional data frames: Using PySpark with JSON data 7 Bilingual PySpark: Blending Python and
SQL code 8 Extending PySpark with Python: RDD and UDFs 9 Big data is just a lot of small data: Using pandas UDFs 10 Your data under a different lens: Window functions 11 Faster PySpark: Understanding Spark’s query planning PART 3 GET CONFIDENT: USING MACHINE LEARNING WITH PYSPARK 12 Setting the stag
e: Preparing features for machine learning 13 Robust machine learning with ML Pipelines 14 Building custom ML transformers and estimators
Learn Aspen Plus in 24 Hours, 2e
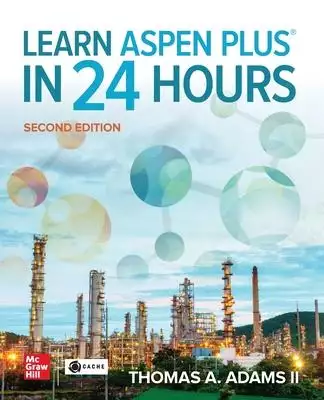
為了解決Quick sort Python 的問題,作者Adams, Thomas A. 這樣論述:
Quickly start using the current version of Aspen Plus(R) to solve chemical engineering problemsDiscover how to solve chemical engineering problems with Aspen Plus(R) in just 24 hours, with no prior experience. Thoroughly revised for the latest distribution, this self-learning guide features detai
led mathematical models for a wide range of chemical process equipment, including heat exchangers, pumps, compressors, turbines, distillation columns, and chemical reactors. Divided into 12 two-hour lessons, Learn Aspen Plus(R) in 24 Hours, Second Edition shows, step by step, how to build process mo
dels and simulations without performing tedious calculations. You will also get downloadable Aspen Plus simulation files and helpful quick starter templates.Inside, you will learn how to: Get up and running with Aspen PlusAccurately model physical propertyWork with Aspen Plus’ problem solving toolsC
reate equilibrium- and rate-based distillation modelsBuild chemical reactor modelsIncorporate connections to Microsoft Excel and Python in your Aspen Plus modelsEstimate capital costsOptimize heat exchanger networksSimulate electrolyte chemistry and CO2 captureEmploy parallel computing and optimizat
ionChoose property packages
想知道Quick sort Python更多一定要看下面主題
Quick sort Python的網路口碑排行榜
-
#1.What is Quick Sort Algorithm: How does it work and its ...
Quicksort is one of the most efficient sorting algorithms. It works by breaking an array (partition) into smaller ones and swapping (exchanging) ... 於 www.simplilearn.com -
#2.14: Improving Quicksort
#demonstrates quick sort with median-of-three partitioning from random import ... in Python, #hence using a convention: name prefixed with an underscore, ... 於 tbc-python.fossee.in -
#3.Hoare's Quicksort Algorithm in Python
Quicksort overview · Quicksort sets the low and high partition indices · Pointer ( i ) travels from low up until pivot < array[i] · Pointer ( j ) travels from high ... 於 csanim.com -
#4.An Overview of QuickSort Algorithm - Towards Data Science
Quicksort is one of the most popular sorting algorithms that uses ... Let's look at quicksort programs written in JavaScript and Python ... 於 towardsdatascience.com -
#5.Quick Sort Algorithm using C , C++, Java, and Python
Quick Sort Pseudocode · We are given with an input array · Choose pivot, here we are choosing the last element as our pivot · Now partition the array as per pivot. 於 www.mygreatlearning.com -
#6.Quicksort using Python | Aman Kharwal - thecleverprogrammer
Quicksort using Python ... Quicksort is a sorting algorithm that selects an item and rearranges the array forming two partitions so that all items ... 於 thecleverprogrammer.com -
#7.How to sort a list using Quicksort algorithms | PyShine
Here is a Python implementation of the classic QuickSort algorithm: def quick_sort(arr): if len(arr) <= 1: return arr pivot = arr[0] less ... 於 pyshine.com -
#8.常見程式演算:: 快速排序(一) - OpenHome.cc
快速排序法(Quick sort)是常用的排序方法之一,精神是分而治之,以升冪為例,基本上是數列中選出作為軸的數字S,將數列分為小於S 的子數列. 於 openhome.cc -
#9.[알고리즘] 퀵 정렬 - Quick Sort (Python, Java)
[알고리즘] 퀵 정렬 - Quick Sort (Python, Java). 이번 포스팅에서는 가장 유명한 정렬 알고리즘 중 하나인 퀵 정렬(Quick Sort)에 대해서 알아보겠습니다. 於 www.daleseo.com -
#10.Quick Sort - 快速排序
Quick Sort - 快速排序. out-in-place - 非原地快排. 复杂度分析. in-place - 原地快排. one index for partition; Python; Java; Two-way partitioning; Python; Java. 於 algorithm.yuanbin.me -
#11.Quick Sort Recursive Python Program - Bytes of Intelligence
Quick Sort is an effective sorting algorithm that makes the use of pivots. It is known as a divide-and-conquer algorithm … Quick Sort Recursive Python ... 於 bytesofintelligence.co.uk -
#12.The Quicksort Algorithm in Python - Finxter
If you don't know either, let's work on the first one! The Quicksort Algorithm — A Python Implementation. 於 blog.finxter.com -
#13.Python Quicksort Algorithm - CodersLegacy
As the name implies, it's a way of quickly sorting a list of values. Typically done with a recursive solution, Quick sort uses the concept of a pivot around ... 於 coderslegacy.com -
#14.Python Program for Quicksort - Studytonight
Algorithm · Create a function partition() · Pass three parameters arr, low, high · Select rightmost element as pivot · declare pointer for greater element · traverse ... 於 www.studytonight.com -
#15.Quick Sort Algorithm | Python - Nodejsera
Implementing QuickSort using Python. What is quicksort and how it works ? QuickSort is an very efficient sorting algorithm based on divide and conquer rule. 於 www.nodejsera.com -
#16.Quick Sort Algorithm - Javatpoint
Quicksort picks an element as pivot, and then it partitions the given array around the picked pivot element. In quick sort, a large array is divided into two ... 於 www.javatpoint.com -
#17.A Python Tutorial on Partition and QuickSort Algorithm
Quicksort In Python · If i<j, increment i and stop when the pivot element is less than ith element. · For i<j, decrement j and stop when the pivot ... 於 www.analyticssteps.com -
#18.QuickSort Algorithm - AlgoTree
Thus giving a worst-case time complexity of O ( n 2 ). Quicksort Implementation. Python C++ Java. 於 www.algotree.org -
#19.Quicksort - CodesDope
Implement quicksort in C, Java and Python with illustrated graphics and examples. Read the clear explanation and codes. 於 www.codesdope.com -
#20.Sample Code - Quick Sort in Python - HijosDeInit
Python program for implementation of Quicksort Sort # This function takes last element as pivot, places # the pivot element at its correct position in ... 於 hijosdeinit.gitlab.io -
#21.Quick Sort - CodeCrucks
Quick sort was invented by Tony Hoare. It is a divide and conquer strategy. Quicksort is sometimes referred to as partition exchange sort. 於 codecrucks.com -
#22.Quick Sort using Python - CherCher Tech
Quick Sort using Python · Step 1 : Consider the array to be sorted quick-sort-1 · Step 2 : Selecting elements lesser than and greater than the pivot element and ... 於 chercher.tech -
#23.Python Quicksort, algoritmo di ordinamento Python
We implement the Python Quicksort algorithm, also known as the sorting algorithm which is based on the divide and conquer approach! 於 www.codingcreativo.it -
#24.Quicksort using divide and conquer method in Python
python program for quick sort algorithm ... In this program, we are going to learn the quicksort algorithm using Divide and conquer approaches/method in Python. 於 www.codespeedy.com -
#25.quick sort - Washington
Quick sort is another divide and conquer algorithm: – Choose one element in the list to be the pivot. – Divide the elements so that all elements less than ... 於 courses.cs.washington.edu -
#26.Lecture 4: Quicksort analysis - NPTEL MOOC
ALGORITHMS IN PYTHON. Madhavan Mukund, Chennai Mathematical Institute ... def Quicksort(A,l,r): # Sort A[l:r] ... Quicksort(A,yellow,r). Quicksort in Python ... 於 www.cmi.ac.in -
#27.Python Program for QuickSort - Tutorialspoint
Here we first partition the array and sort the separate partition to get the sorted array. Now let's observe the solution in the implementation ... 於 www.tutorialspoint.com -
#28.12 Quick Sort - NeetCode
12 - Quick Sort. Get Unlimited Access to Videos. Mark Lesson Complete ... Sort An Array. Medium. Kth Largest Element In An Array. Medium. Python ... 於 neetcode.io -
#29.Quick Sort Python - Linux Hint
Quicksort is a well-liked sorting algorithm that is frequently used. Quicksort represents divide-and-conquer, in-place, and unstable sorting algorithms. 於 linuxhint.com -
#30.How to Implement Quick Sort in Python? - Coding Ninjas
QuickSort is a sorting algorithm that is widely used in the industry. It is fast, efficient, and powerful. It uses the divide-and-conquer technique to ... 於 www.codingninjas.com -
#31.Implementing Quicksort in Python | TutorialEdge.net
QuickSort Algorithm · A Random Pivot element is chosen from our unsorted array. · We create 3 distinct partitions : · We iterate through our array ... 於 tutorialedge.net -
#32.QuickSort Complete Tutorial | Example | Algorithm | Complexity
How does QuickSort work? QuickSort Algorithm; QuickSort Programming. C/C++ Programming; Python Programming; Java Programming. QuickSort Time Complexity ... 於 www.csestack.org -
#33.Quick Sort | Sololearn: Learn to code for FREE!
Quick Sort : Write a program that sorts an array by selecting a pivot, putting it in its right place, with all smaller elements on ... Introduction to Python. 於 www.sololearn.com -
#34.【演算法筆記#3】QuickSort 重點整理筆記,包含Partition ...
QuickSort 算是面試中或是演算法中經典的問題,非常重要, 而且最容易搞錯「他的範圍」,因此這 ... 內含:python sorted key 搭配lambda 的用法範例. 於 www.wongwonggoods.com -
#35.Quicksort Algorithm in Python - Koder Dojo
Quicksort is an in-place sorting algorithm. Therefore, when you pass it an array of integers, for example, it will mutate that array and sort it in increasing ... 於 www.koderdojo.com -
#36.Python Quick Sort Program
We will write a function named quick_sort() which takes list as argument and sorts this list using Quick Sort algorithm. Also, by default, this quick_sort() ... 於 pythonexamples.org -
#37.Quick Sort in C++ ( Code with Example) - FavTutor
Understand what is quick sort algorithm and its c++ code. Also, learn about quicksort time complexity and its example. 於 favtutor.com -
#38.Quick Sort in Python - eduCBA
Quick sort algorithm is an in-place sorting algorithm without the need of extra space or auxiliary array as all operations will be done in the same list, as we ... 於 www.educba.com -
#39.[演算法] 快速排序法(Quick Sort) - iT 邦幫忙
快速排序(Quick Sort) 的想法是說,先找一個基準點,然後派兩個代理人分別從資料的兩邊開始往中間找,如果右邊找到一個值比基準點小,左邊找到一個值 ... 於 ithelp.ithome.com.tw -
#40.Python 快速排序 - 菜鸟教程
Python 快速排序Python3 实例快速排序使用分治法(Divide and conquer)策略来把一个序列(list)分为较小和较大的2个子序列,然后递归地排序两个子序列。 於 www.runoob.com -
#41.Sorting algorithms/Quicksort - Rosetta Code
Task Sort an array (or list) elements using the quicksort algorithm. The elements must have a strict weak order and the index of the array can be of any... 於 rosettacode.org -
#42.6.12. The Quick Sort - Runestone Academy
The quick sort uses divide and conquer to gain the same advantages as the merge sort, while not using additional storage. As a trade-off, however, ... 於 runestone.academy -
#43.Bubble Sort Merge Sort and Quick Sort in Python - vegibit
A bubble sort starts by comparing the first two elements to each other to see which is larger. If the first element is bigger than the second, then the two ... 於 vegibit.com -
#44.(PDF) Multi-Pivot Quicksort: an Experiment with Single, Dual ...
In this research, we implement and experiment with single, dual, triple, quad, and penta-pivot quicksort algorithms in Python. 於 www.researchgate.net -
#45.The Complete Quick Sort Guide - Crio.Do
So, no action is performed. Implementation of Quick Sort in Python. # The main function that implements QuickSort def quicksort(array, l, r): if ... 於 www.crio.do -
#46.A naive Quicksort in Python - gists · GitHub
A naive Quicksort in Python. GitHub Gist: instantly share code, notes, and snippets. 於 gist.github.com -
#47.QuickSort (With Code in Python/C++/Java/C) - Programiz
Quicksort is an algorithm based on divide and conquer approach in which an array is split into sub-arrays and these sub arrays are recursively sorted to get ... 於 www.programiz.com -
#48.Quick Sort | Discovering Python & R
In my earlier post on the Python code for Quick Sort, my implementation takes the first element of the unsorted array as the pivot element. However with some ... 於 pythonandr.com -
#49.How to Implement QuickSort in Python? - AskPython
Quicksort is a sorting algorithm that follows the policy of divide and conquer. It works on the concept of choosing a pivot element and then ... 於 www.askpython.com -
#50.python实现·十大排序算法之快速排序(Quick Sort) - SegmentFault
快速排序(Quick Sort)是对冒泡排序的一种改进,其的基本思想:选一基准元素,依次将剩余元素中小于该基准元素的值放置其左侧,大于等于该基准元素的值 ... 於 segmentfault.com -
#51.Quick Sort Algorithm Explained (Full Code Included) - YouTube
Quick Sort Algorithm Explained (Full Code Included) - Python Algorithm Series for Beginners. 於 www.youtube.com -
#52.Python Program for Quick Sort - AlphaCodingSkills
Quick sort is a divide and conquer algorithm. It is based on the idea of choosing one element as a pivot and partitioning the array around the pivot with ... 於 www.alphacodingskills.com -
#53.In place QuickSort algorithm in Python
Fix your function signature. Most Python programmers use lowercase for function names. Even more important, don't swap the order the left ... 於 codereview.stackexchange.com -
#54.Quicksort - Wikipedia
Quicksort is an efficient, general-purpose sorting algorithm. Quicksort was developed by British computer scientist Tony Hoare in 1959 and published in 1961 ... 於 en.wikipedia.org -
#55.Python Tutorial 19 | Quick Sort(快速排序法) - 阿嬤
Python Tutorial 19 | Quick Sort(快速排序法). 前言. 只要你懂排序法的原理,排序法程式就會幫助你。 原理 ... 於 anchi-tang.medium.com -
#56.Quick Sort Algorithm in Data Structures - Scaler Topics
Quick sort in Python # finding the partition point & rearranging the array def partition(array, low, high): # selecting the rightmost ... 於 www.scaler.com -
#57.Quick Sort Algorithm - 101 Computing
Python Code · #Quick Sort Algorithm - 101computing.net/quick-sort-algorithm · #Initialise the Pivot using the element in middle position of the ... 於 www.101computing.net -
#58.Quicksort with Python - algorithm - Stack Overflow
Quicksort is not very practical in Python since our builtin timsort algorithm is quite efficient, and we have recursion limits. We would expect to sort lists in ... 於 stackoverflow.com -
#59.How to implement QuickSort in Python - Educative.io
QuickSort is an in-place sorting algorithm with worst-case time complexity of n 2 n^{2} n2. Implementation. QuickSort can be implemented both iteratively ... 於 www.educative.io -
#60.Quick Sort in C » PREP INSTA
Learn to code with PrepInsta ... Learn from blogs on PrepInsta to check Top 100 Codes, C, C++, Java, Python, DSA Competative Coding and more ... 於 prepinsta.com -
#61.Python Program for QuickSort - GeeksforGeeks
Just unlikely merge Sort, QuickSort is a divide and conquer algorithm. It picks an element as a pivot and partitions the given array around ... 於 www.geeksforgeeks.org -
#62.Quick sort - Isaac Computer Science
Quick sort, as its name implies, is one of the fastest sorting algorithms. ... The quick sort method sorts a list by selecting a pivot value. 於 isaaccomputerscience.org -
#63.How to Code a Python QuickSort - Career Karma
A Python QuickSort algorithm divides an array into sub arrays. This algorithm calls these sub arrays recursively to sort each element in the ... 於 careerkarma.com -
#64.Quicksort in Python - Stack Abuse
Divide and conquer: Quicksort splits the array into smaller arrays until it ends up with an empty array, or one that has only one element, ... 於 stackabuse.com -
#65.It took me hours to understand the quick sort algorithm ... - Quora
Quick sort is a general sorting algorithm that puts a list of items in ascending or descending order. Python has no built in quick sort function, so you would ... 於 www.quora.com -
#66.Python quick sort solution easy to understand beats 95.51%
Python quick sort solution easy to understand beats 95.51%. feather. 85. Mar 09, 2016. To solve the TLE problem, I set two pivots in the partition function, ... 於 leetcode.com -
#67.『QuickSort』详解「快速排序」的Python实现
Python program for implementation of Quicksort Sort def partition(arr,low,high): i = ( low-1 ) pivot = arr[high] for j in range(low , high): if arr[j] ... 於 chihokyo.com -
#68.Quicksort In Python Explained (With Example And Code)
Quicksort is an efficient sorting algorithm with O(n*logn) average running time. In this video I show you a quick example and how to ... 於 www.youtube.com -
#69.Quick Sort - Python - GlobalSQA
Quicksort can then recursively sort the sub-arrays. The steps are: Pick an element, called a pivot, from the array. Partitioning: reorder the ... 於 www.globalsqa.com -
#70.Quick Sort - Study Notes
If the input is randomly arranged, then the left and right sublists will also be random. Implementations. Python. from random import shuffle def ... 於 www.studies.nawaz.org -
#71.Quicksort algorithm in Python (Step By Step) - Like Geeks
In the Quicksort algorithm, the partitioning is done in place. This requires O(1) space. The elements are then recursively sorted and for each ... 於 likegeeks.com -
#72.QuickSort Algorithm (With Code) - Naukri Learning
In this blog, we will explore what is quicksort algorithm with example, its implementation in C and Python, and its time & space complexity. 於 www.naukri.com -
#73.Quick Sort: A Tutorial and Implementation Guide
Here's a simple and easy tutorial to learn how to sort using Quick Sort, and learn about its algorithm and its implementation in Python. 於 www.pythoncentral.io -
#74.Python Sorting Algorithm: Quick Sort | by Abel Garrido
Quick -sort is another divide and conquer algorithm like the merge_sort algorithm. Quick-sort's unique method however makes it oddly ... 於 python.plainenglish.io -
#75.快速排序(Quick sort)Python实现 - CSDN博客
3. 对这两个子数组进行快速排序。 Python代码如下:. def quickSort(array):. if len(array)<2: return array. else: pivot=array[0] #选择基准值. 於 blog.csdn.net -
#76.QuickSort - A python example
#!/usr/bin/env python # Written by Magnus Lie Hetland "Everybody's favourite sorting algorithm... :)" def partition(list, start, end): pivot = list[end] ... 於 folk.idi.ntnu.no -
#77.Sorting Algorithms in Python
Just like merge sort, the Quicksort algorithm applies the divide-and-conquer principle to divide the input array into two lists, the first with small items and ... 於 realpython.com -
#78.Quick Sort Algorithm in Data Structures - W3schools
Quick sort is one of the most famous sorting algorithms based on divide and conquers strategy which results in an O(n log n) complexity. 於 www.w3schools.in -
#79.Quick Sort Algorithm Explained (Full Code Included) - Python ...
The Quick Sort algorithm takes an item from the unsorted list and uses it as the 'pivot' or the item to compare the remainder of the items in the list. We do ... 於 www.derricksherrill.com -
#80.Python Quick Sort - The Crazy Programmer
Here you get python quick sort program and algorithm. Quick sort is based on divide and Conquer technique. We divide our array into sub-arrays and that ... 於 www.thecrazyprogrammer.com -
#81.QuickSort in Python - STechies
QuickSort implementation in Python ... Output: Explanation: First we create a user-defined function to find the partition position and pass three parameters ... 於 www.stechies.com -
#82.Showing Off Quicksort in Three Lines - Python Cookbook [Book]
Showing Off Quicksort in Three Lines Credit: Nathaniel Gray Problem You need to show that Python's support for the functional programming paradigm is quite ... 於 www.oreilly.com -
#83.Quicksort - divide and conquer - Java Python JavaScript
Quicksort uses divide and conquer technique to provide fast sorting. Code is available in Java, JavaScript and Python. 於 www.lavivienpost.net -
#84.Python: Quick sort - w3resource
Write a Python program to sort a list of elements using the quick sort algorithm. Note : According to Wikipedia "Quicksort is a comparison sort, ... 於 www.w3resource.com -
#85.Quicksort Algorithm – C++, Java, and Python Implementation
Quicksort is an efficient in-place sorting algorithm, which usually performs about two to three times faster than merge sort and heapsort when implemented well. 於 www.techiedelight.com -
#86.Quick sort - Python - OneCompiler
def quicksort(arr,left,right): if left<right: ... quicksort(arr,pivot+1,right) ... Getting started with the OneCompiler's Python editor is easy and fast. 於 onecompiler.com -
#87.[Python] Quick sort - Hubert's Coding Notes
[Python] Quick sort. Algorithms、Python / 2022 年4 月11 日 2022 年4 月11 日. def quick_sort(nums, left=None, right=None): def _partition(nums, left, right, ... 於 www.nyanblock.com -
#88.Quicksort tutorial: Python implementation with line by line ...
A reference Quicksort implementation with an intuitive explanation as well as a line-by-line breakdown. This tutorial will get you unstuck ... 於 www.codementor.io -
#89.Quick Sort - Data Structures & Algorithms Tutorial Python #15
Quick sort is a popular sorting algorithm invented by British scientist Tony Hoare. Often interviewers ask questions around quick sort in ... 於 www.youtube.com -
#90.Quicksort Python Program - CSVeda
Quicksort follows divide and conquer technique to sorting of a set of elements. Checkout Quicksort Python code with list as stack to store ... 於 csveda.com -
#91.Learn Sorting Algorithms with Python: Quicksort Cheatsheet
Quick Sort General. Quicksort is a method for sorting an array by repeatedly partitioning it into sub-arrays by: Selecting an element from ... 於 www.codecademy.com -
#92.QuickSort on Python - Level Up Coding
What is Quicksort? ... From what I learnt yesterday, quick sort involves a randomly assigned pivot being chosen in a list and then the values of ... 於 levelup.gitconnected.com -
#93.An Array-Based Implementation of Quick-Sort
Since quicksort is recursive, we have to increment the recursion limit of Python, because otherwise we would get an error that we exceed the maximum recursion ... 於 notebook.community -
#94.Arrays - InterviewBit
Sort array with squares! ... Fast Track Courses · Python · Java · C++ · Javascript. Online Interviewbit Compilers. Online C Compiler · Online C++ Compiler ... 於 www.interviewbit.com -
#95.[ 資料結構] 快速排序法(Quick sort)in Python - Jialin - 痞客邦
快速排序法(Quick sort)運用到Divide and conquer 的概念, 把數列一分為二,最終完成排序。 步驟為: 1. 取第一個元素(最左的數)為鍵值key. 於 jialin128.pixnet.net -
#96.Quick Sort | CS240 Fall 2014
Quick Sort Implementation def quick_sort(items): n = len(items) ... Sort the provided Python list using in-place quick sort.""". 於 w3.cs.jmu.edu -
#97.The Quick Sort - Andrea Marino
The quick sort uses divide and conquer to gain the same advantages as the merge sort, while not using additional storage. As a trade-ÁÁ off, however, ... 於 www.andreamarino.it -
#98.Quick Sort Algorithm In Data Structures And Algorithms Using ...
The complete working of the Quick Sort Algorithm with pseudocode, main code, features, applications, FAQs using python language. 於 copyassignment.com -
#99.Python Program to Implement Quick Sort - Sanfoundry
Python Program to Implement Quick Sort · 1. Create a function quicksort that takes a list and two variables start and end as arguments. · 2. If end – start is not ... 於 www.sanfoundry.com